Manufacturing Analytics – A Simple Guide for SMEs
Manufacturing analytics is the process of collecting and analyzing data to get insights for improving efficiency in a manufacturing organization. While MA used to be the playing field of only large enterprises, nowadays even the smallest manufacturer can benefit from it.
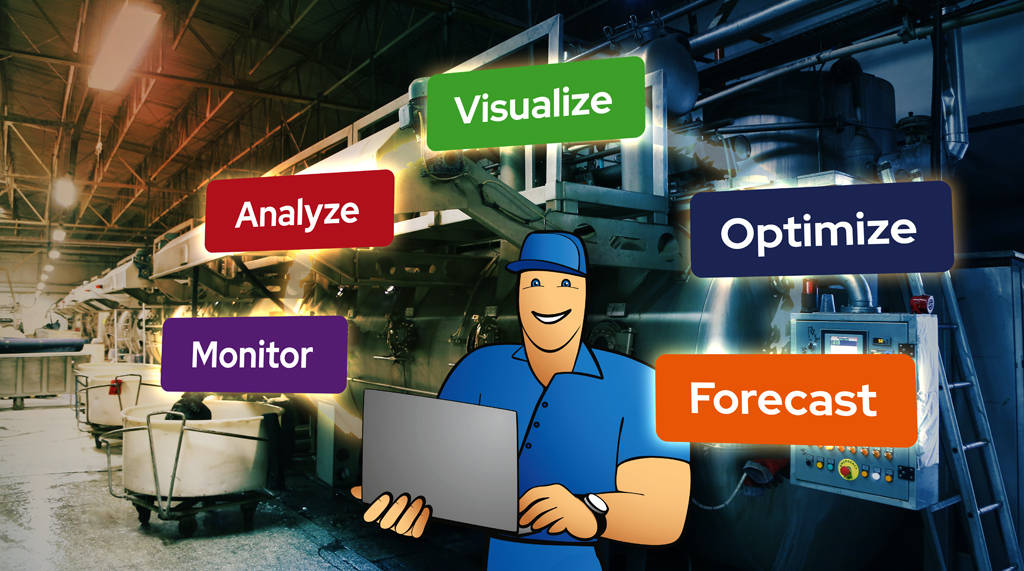
What is manufacturing analytics?
Manufacturing analytics is the process of collecting, analyzing, and interpreting data related to a manufacturing operation. This is done in order to gain valuable insights that would help improve the performance of different variables in the manufacturing process and the operation as a whole, predict potential issues before they become actual problems, and react quickly to unpredicted events.
Manufacturing analytics can either be viewed as dealing specifically with physical production processes or as an umbrella term for all processes that facilitate the production of goods. In the latter sense, manufacturing analytics also includes areas such as inventory management, procurement, sales, and finances. In this article, we will use the wider term to approach MA.
Although advanced manufacturing analytics belongs to the realm of complex data science and is rarely reachable for small manufacturers, MRP/ERP software is a good start for manufacturers of any size.
Benefits of manufacturing analytics
Manufacturing analytics offers an array of benefits, which is why it is becoming increasingly important not only in large enterprises but in small businesses as well. Some of the key benefits of manufacturing analytics are:
- Improved efficiency. Manufacturing analytics provide you with actionable insights to improve processes across your business and supply chain. For example, measuring and analyzing your data allows you to detect bottlenecks and reduce downtime on the shop floor, determine your ideal inventory levels, identify demand patterns and underperforming products, assess supplier performance, etc.
- Reduced costs. Every little improvement in production efficiency translates into a reduced cost. Having a precise overview of your inventory and accurately predicting demand allows you to avoid stockouts and overstocking, thereby minimizing inventory costs. Determining optimal lot sizes enables you to order less frequently, reducing ordering costs. Manufacturing analytics helps companies streamline their operation and minimize costs across the board, resulting in improved profitability.
- Enhanced product quality. Analyzing production and quality control data can help you discover inconsistencies, find the root causes of issues, and take corrective actions. This will result in fewer defects, scrap, and rework.
- Better decision-making. Data analysis enables you to make informed decisions that lead to better business outcomes than relying on guesswork. For example, accurate forecasting results in better production planning and inventory management, ample production data shows you exactly which areas need improvement or which employees need extra training, real-time cost analysis enables you to choose the best supplier, etc.
- Increased customer satisfaction. Increasing the efficiency of your manufacturing operation will sooner or later reflect in higher customer satisfaction. In addition, being able to analyze and interpret data in real time enables you to communicate quicker and more clearly with your customers at any stage of the order fulfillment process.
- Continuous improvement. Manufacturing analytics enables SMBs to develop advanced continuous improvement strategies that take advantage of improved decision-making, quality, and efficiency gains. This cements improvement into a culture and makes SMBs more competitive.
Manufacturing analytics can help you attain all of the benefits above, thereby allowing you to gain a sharp competitive advantage over other, less data-driven businesses.
Types of manufacturing analytics
To better understand the various ways to approach manufacturing analytics, let’s divide it into different types.
Basic vs. advanced analytics
Basic and advanced manufacturing analytics differ in their complexity, the tools used, and the level of insight they provide. While basic MA most often utilizes spreadsheets and basic algorithms to collect and process data, advanced manufacturing analytics requires the use of more sophisticated tools, such as MRP/ERP software, sensors, and other IIoT (Industrial Internet of Things) devices, even artificial intelligence and machine learning.
The value of basic analytics should not be understated as it provides plenty of information to companies that are just starting out. As a business matures, however, its capacity to understand and make use of analytics grows, and at some point, it is time to look for more advanced solutions.
Descriptive vs. diagnostic vs. prescriptive vs. predictive analytics
These four types of data analytics differ by their output. Descriptive analytics produces an overview of a situation, diagnostic analytics determines the root cause of an issue, prescriptive analytics proposes a course of action, and predictive analytics aims to forecast future outcomes.
Descriptive analytics uses data to describe a situation, to define what has happened and when. This includes capacity and material requirements planning, identifying sales trends, discovering bottlenecks, analyzing costs, assessing the performance of various departments and factors, etc. Descriptive analytics is the basis for identifying changes in a process and understanding how the changes affect the outcome. Descriptive analytics tools are widely present in business management systems such as MRP/ERP software.
Diagnostic analytics is the next step from descriptive analytics. When an issue has been discovered, diagnostic analytics is used to identify the root cause of the problem. While descriptive analytics finds out what happened and when, diagnostic analytics finds out why something happened. Even though there are some designated analytics platforms out there that use machine learning and artificial intelligence, they are most often too expensive for small manufacturers. Instead, small businesses can use their data and perform root cause analysis manually to figure out the core of an issue.
Prescriptive analytics aims to propose possible solutions to the diagnosed issue. As diagnostic analytics tools, prescriptive analytics software uses new technologies like machine learning and AI with advanced algorithms that allow the tool to make decisions based on statistics and patterns.
Predictive analytics relies on historical and real-time data in order to predict what is going to happen. Use cases include predictive maintenance, equipment failure predictions, and demand forecasts. Predictive analytics also relies on advanced tools such as IoT sensors, machine learning algorithms, and statistical analysis software.
Common problems related to manufacturing analytics
Although manufacturing analytics, when properly used, can facilitate optimization and problem-solving across your company, it can also complicate matters if done poorly. These are some of the common pitfalls of manufacturing analytics:
- Data siloing. Data siloing happens when different departments (or even different employees) keep data in separate systems. For example, when manufacturing data is kept on paper-based media, inventory data in computer spreadsheets, sales data in a CRM system, etc. When data connectivity is lacking, manufacturing companies cannot take full advantage of analytics regardless of the amount of data they collect and process.
- Bad data. The concept of garbage in, garbage out (GIGO) specifies that the outputs of a system are only as good as the inputs. This means that if the data inputs are inaccurate, the outputs of the analytics system are also inaccurate, making them unusable. Always verify the correctness of the data that you put into a data processing system.
- Not using proper tools. Using spreadsheets to collect and analyze big data is a bad idea as the risk of errors and the unscalability of manual systems become huge impediments sooner or later, not to mention the vast amount of time data entry would eat up. The correct first step in manufacturing analytics is to implement MRP/ERP software which would act as the infrastructure for information processing and sharing.
- Resource-heavy tools. While modern MRP/ERP software is affordable and easy to use for manufacturing professionals, adopting more advanced solutions such as IIoT and dedicated analytics platforms is an expensive endeavor and requires a lot of knowledge to navigate the data.
Regardless of the drawbacks, every manufacturer that is looking to grow should take steps to implement manufacturing analytics.
Implementing manufacturing analytics in a small business
Adopt MRP/ERP software
MRP/ERP should be the first analytics solution implemented within a manufacturing company as its importance lies not only in analytics but also in organized data collection and processing which significantly reduces the risk of data entry errors.
This type of software integrates the whole company, allowing for effective cross-departmental collaboration and the optimization and automation of an array of processes. Simplifying tasks from production planning to purchasing, from quoting to fulfillment, MRP/ERP software reduces the huge managerial and administrative workload of the manufacturing industry and enables companies to focus on growing the business.
The software also provides small manufacturers with essential analytical capabilities such as capacity planning, raw material planning, production scheduling, cost analysis, KPI tracking, some data visualization, etc. By providing instant access to historical data as well as real-time information, MRP/ERP facilitates processes such as bottleneck detection, root cause analysis, and demand forecasting.
Although traditionally, MRP/ERP software has been unavailable for small manufacturers due to its pricing and complexity, modern cloud-based solutions are so affordable and user-friendly that even micro companies use them.
Track vital KPIs
There are dozens or hundreds of KPIs small manufacturers may track. That is why it is feasible to choose a handful of metrics that are most important to your business. Here are some KPIs worth your consideration:
- Overall Equipment Effectiveness. OEE measures the availability, performance, and output quality of specific pieces of equipment or production lines.
- Total Effective Equipment Performance. Similar to OEE, TEEP measures the availability, performance, and output quality of equipment. The difference from OEE is that TEEP measures efficiency in relation to all calendar time, not only planned shift time.
- On Time, In Full. OTIF measures the percentage of orders that have been fulfilled on the scheduled date and with the full ordered quantity.
- Cost per Unit. CPU shows the total cost of manufacturing a single unit of a product.
- Rejection rate. The rejection rate measures the percentage of defective products from the total. You can also use the first-time yield to measure the percentage of products without defects.
- Manufacturing on time. MOT shows the percentage of orders or production runs completed on schedule.
- Purchases on time. POT shows the percentage of purchases that have been delivered on time. Also measure POT per supplier to assess vendor performance.
- Inventory turnover ratio. Inventory turnover rate shows the number of times you sell and replace your inventory over a year.
- Lead time. Lead time is the time it takes to fulfill an order. By breaking it down, you can discover inefficiencies in the process.
- Work-in-process inventory. WIP measures the value of products that are in the process of being manufactured at any given time.
- Cycle time. Cycle time is the time it takes to complete one production step, e.g. the assembly of a table.
- Throughput time. Throughput time is the time it takes for a product to flow through the whole value-added manufacturing process.
Some MRP/ERP systems allow you to create a custom dashboard where you can keep an eye on your company’s vital metrics all in one place.
Read more: Top 10 Manufacturing KPIs
Perform root cause analysis
While MRP/ERP provides you with an array of descriptive analytics insights, root cause analysis is used to turn descriptive analytics into diagnostics. In simple terms, MRP/ERP shows you where the problem is and root cause analysis will determine why the problem has arisen.
There are several approaches to root cause analysis, ranging from very simple methods like the Five Whys to a little bit more complex ones like the Fishbone and Matrix Diagrams.
Using root cause analysis allows you to set out on a path to continuous improvement without spending money on expensive analytics systems.
Read more: Root Cause Analysis in Manufacturing.
Add solutions as you grow
When you have fully implemented an MRP/ERP software and still feel that you need to dive deeper into the world of manufacturing analytics, it is time to look for more advanced smart manufacturing solutions.
These may include designated analytics platforms that allow you to further analyze the data collected by the MRP/ERP system, sensors and other IIoT solutions that allow for remote monitoring and predictive analytics, digital twins that simulate processes and help you find ways to optimize them, etc.
Make sure you have the resources and capacity to implement further solutions as these come with a substantially bigger learning curve compared to MRP/ERP.
Key takeaways
- Manufacturing analytics is the process of collecting, analyzing, and interpreting data related to a manufacturing operation in order to gain valuable insights that would help improve performance, predict potential issues, and react quickly to unpredicted events.
- Analytics are generally divided into four groups: descriptive (what happened), diagnostic (why it happened), prescriptive (what to do about it), and predictive diagnostics (what is likely to happen).
- Common drawbacks when using manufacturing analytics are data siloing, low-quality data, using sub-par tools, and the lack of resources for implementing analytics.
- In a small manufacturing business, the first step toward implementing manufacturing analytics would be to adopt MRP/ERP software.
Frequently asked questions
Analytics in manufacturing involves the use of data analysis and statistical methods to increase efficiency, reduce costs, and prevent issues in a manufacturing company.
The four types of analytics are descriptive analytics (what happened), diagnostic analytics (why it happened), predictive analytics (what is likely to happen), and prescriptive analytics (what action to take).
The benefits of analytics in manufacturing include improved quality control, increased efficiency, reduced costs, better decision-making, improved customer satisfaction, and continuous improvement.
Manufacturing can be analyzed by collecting and analyzing data on various aspects of the production process, including equipment performance, quality control, supply chain management, and inventory management, using statistical and data analysis techniques.
You may also like: Frozen Food Manufacturer Raises Efficiency by 400%